Let’s have a look at the early concept which will define what data to preserve and what you should ditch
In 2006, British mathematician and Tesco marketing mastermind Clive Humby shouted from the rooftops, “Data is the new oil.” Some say data is the new gold.
This has led to lunatic ideas to horde data. Logic often is that more data is more value, we might have some value in it so let's gather it all and store it in the cloud. Sounds a bit like the after-effects of big data hype. That is as crazy as hoarding toilet paper to survive covid-19. In fact, it's even crazier.
Toilet paper and gold keep the value better than the significant share of data stored.
The phenomenon we are witnessing is the data value half-life. Jarkko Moilanen coined the term in the Data Mesh radio podcast in the spring of 2022. Besides gold and oil are stable and do not have half-time. In that sense, data is more like a radioactive isotope. The concept of half-life is borrowed from physics and should not be taken literally, but rather as a well-known model of the decay process.
While there is no exact formula (yet) to draw a mathematical curve of data value half-time it’s something we can already now witness in real life. According to it, some data retain their value for a significantly long time.
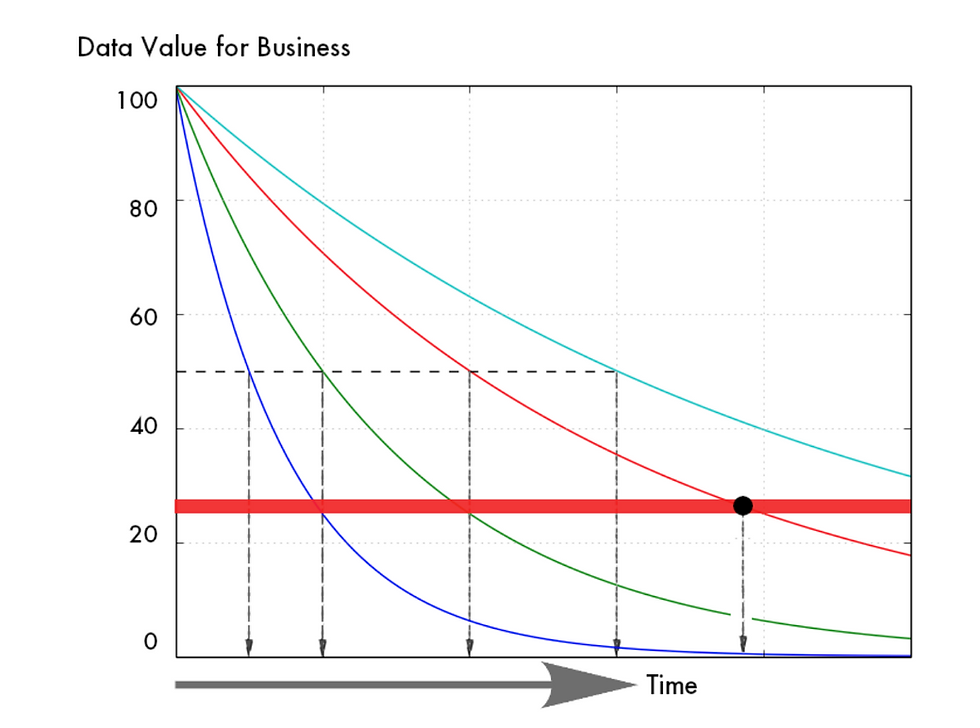
When the above is described with illustration it could be something like the above. Colored lines describe the behavior of imaginary data. Some decay faster, some slower. Data probably always has some value, but at some point in time business value of data is less than the costs of keeping it. This raises a couple of questions to consider: when is the optimal moment to pull the value from data, move it to lower-cost storage, delete it, and when does it cross the border of causing more costs than benefits.
Watch the video or continue reading
Let’s take some examples!
DNA data is probably such. It can be used for years in medical development. Data is more and more used as an input for systems, whether those are purely machine-driven or hybrids. Regardless of details, this kind of control data often has a significantly shorter value half-time compared to for example the DNA data.
On the other end of the spectrum is for example robots working in a chain to create value. Those have little value to knowing neighboring robots’ statuses 1 or 2 seconds ago. Those machines react and operate in milliseconds to act efficiently. It is obvious that data is losing value occasionally very rapidly.
What are the time-based factors causing data half-life?
According to PwC the more timely and up-to-date the data is, the more valuable it is. This would suggest that timely erosion also affects the value. As an example, data which describes an individual’s location at a precise moment in time is significantly more valuable to a retailer than data that describes their location an hour ago.
The more unique the dataset, the more valuable the data is. Over time competition often emerges and your uniqueness in the markets is challenged. Naturally, when the customer can find alternative data similar to yours from the market, the less valuable your data becomes.
A slightly different approach is to use the willingness to pay as a possible cause of half-time. This is related to the availability of data on the markets. As long as similar data is not available as open data with necessary rights for business purposes, the willingness to pay is higher. But the moment when free options emerge, the willingness to pay lowers.
Comments