I've used the Data Product Toolkit in over 100 cases now and based on the experience it just works. As always, things could be better and thus I crafted a new version of the Data Product Canvas. It became fundamentally different compared to the current version and thus version suggestion is 2.0. The canvas candidate (WIP) is below, click it to enlarge.
Suggested changes to Data Product Canvas based on 100+ cases.

Strategy & Portfolio
At the top is Strategy and Portfolio stream. This is still under heavy development and requires more thorough thinking since customers have had difficulties seeing or connecting one data product under work as part of the bigger portfolio.
At the same time, customers have in many cases started to sketch multiple data product candidates in parallel. In other words, customers create solution sketches for multiple use cases and problems but do not always understand that they are defining possible data product portfolios.
Data products are also part of some bigger business goals and are often beneficial to connect with some business objective as part of your data and more general business strategy.
Data Value Chain
The 2nd stream is the more traditional data value chain. It is pretty much the same as in the previous version. Small adjustments have been made though. The idea is still the same - on the left is the data source(s) and the more right you go the more you are in the customer space.
What has been added there is a separate box for data processing which was not there so clearly before, In many cases data processing is something that the data product owner is not capable of doing. In some cases, a data product is a combination of two or more data streams and thus some processing is needed. Since it is so fundamental piece of the data value chain in practice, I decided to add a box for it in the canvas.
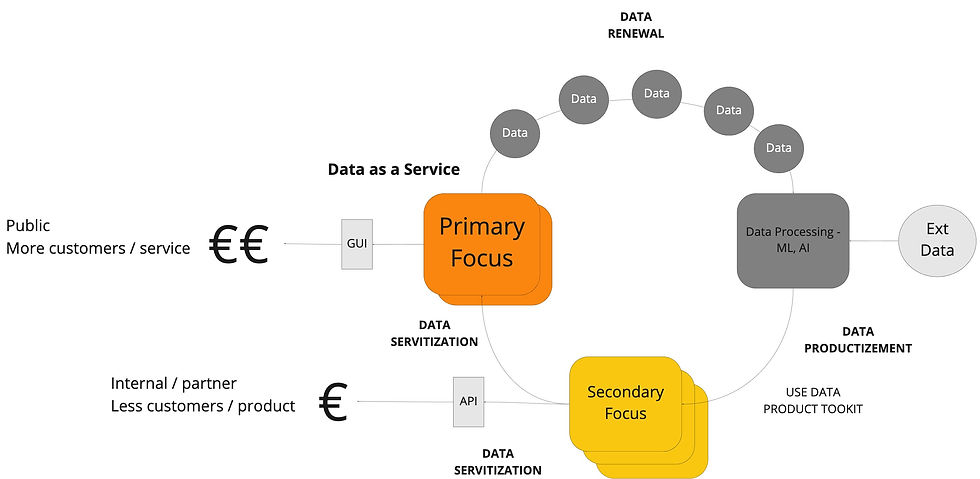
Also, the far right box has been altered. It is renamed to "Supported Customer Environments". The experience has shown us that the more clearly we can name the environments in which data product is used, the more clear it is for all. As an example some data products are aimed only for PowerBI environments while some are aimed for customers using Snowflake environment and ecosystem. The more clearly we know the target environment, the more easier we can make it for customer to engage with the data product. In other words, we can focus on the customer experience in more details.
Legal & Ethical & Customer
Issues related to legal and ethical aspects have now separate swimlane in the canvas. Based on the experiences checking the IP rights for data to be used is something that must be clearly there. Not all source data is in-house production and we must be aware of the limitations we have regarding purchased data. We might not be allowed to mix it or sell it in any format. This is commonly and shortly the intellectual property aspect of source data.
The other fundamentally important aspect is personal data. I've named that part now "data privacy" and it does include all the shades of personal & mydata aspects. The data product owners have the duty to respect the privacy and rights of the people whom the data concerns. Ethical use of data is something that has become more and more important during the last couple of years. The legal checklist is actually one of the additional supportive tools under development to help data productizement.
In the lane, I have also added "Developer eXperience" (DX). This is serving the current target of the data products. Those are build for data scientists and alike who are pretty technical and need to know how to use the product as easily as possible. Thus we need to take Developer eXperience seriously. This is comparable to API Economy, in which DX has been found to be the key element of success in monetizing APIs.
Business & Ecosystem
The bottom lane is also changed a little bit. We have discovered interesting phenomenon among the customers. Most of them still approach data monetization more or less traditional in-house product development and sales. They analyse the data and package it for sales and that's about it.
Some are now taking a different course. Some companies are taking the ecosystem approach. In it, the data product and portfolio of data products are planned in cooperation with other companies. For example, there might be a small closed ecosystem of a data platform company, data owner companies and a ML/AI company. They together work towards a shared goal - co-owned data products.
Instead of one buying services from others, they are all more or less equal and the setting does not remind the old fashion subcontracting at all. Thus it became obvious that we need to put "ecosystem" box in the data product canvas model as well.
This change in data product crafting has effects on the revenue box as well. In the old days of Osterwalder you would just think of the revenue streams. Now you end up discussing revenue sharing models with your ecosystem partners. In some cases, it has got so far that ecosystem partners plan to establish co-owned companies to run the data product business.
Development continues
I explained briefly how I see the next version of the Data Product Canvas. Would you be interested to share your thoughts on it? Or try it with us? Leave a comment or contact us!
Comentários